Self-assembly and self-organization in cell biology
In our research group we study dynamical systems in biology, both in and out of equilibrium. We address questions related to control of self-assembly in the cell, which has to occur at the right time and place for proper function. We are especially fascinated by clathrin-mediated endocytosis, viral exit from cells, and DNA transcription and maintenance. Our multidisciplinary group tackles questions both on principles and design of self-assembling systems, as well as biological function in cells. To study these complex systems, we simulate networks of many interacting components, and construct simplified models that are amenable to analytical theory, with foundations in statistical mechanics. We use and develop modeling techniques from physics, chemistry, and engineering, including molecular models for thermodynamics, reaction-diffusion models for stochastic dynamics in cells, and continuum models for coupling to mechanics. We collaborate frequently with experimental groups.
Spatial and temporal dynamics of vesicle formation in clathrin-mediated endocytosis
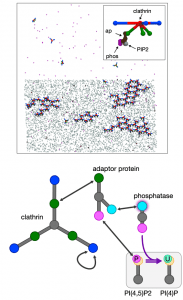
Clathrin-mediated endocytosis is occurring persistently within our cells, performing transport of essential nutrients such as iron into our cells, and clearing receptors at our neural synapse. By controlling the receptor composition at the membrane surface, endocytosis dynamically responds to signals, impacting cell state and motility. It involves coordinated assembly of dozens of different protein components, and viral infection or neurological disorders can result from its dysfunction. Our research has shown how localization to the membrane can dramatically enhance assembly of even very weakly interacting partners via dimensionality reduction from 3D to 2D, which effectively concentrates components and promotes binding. At the systems level, we have quantified how the stoichiometry of the network of protein and lipid components involved in CME can sensitively tune the speed or success of vesicle formation, highlighting again the central role of membrane localization in triggering protein assembly. With reaction-diffusion simulations, we have quantified how essential lipid phosphatases are capable of driving disassembly of the membrane-bound assemblies. Ongoing research in our lab is focused on predicting how the membrane composition and environment controls the frequency and success of vesicle formation for productive receptor uptake and healthy cell function. NIH MIRA
Viral assembly, exit, and membrane remodeling
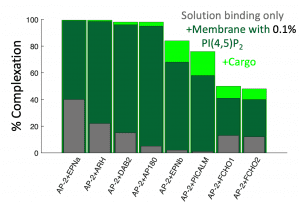
For entry in and exit from the cell, remodeling of the membrane is almost universally driven by self-assembling proteins. To understand the mechanics and dynamics of this remodeling as it couples to the largely biochemically driven interactions between proteins, we develop continuum membrane models and apply them to a range of processes in cell biology (Fu, 2021) (Fu, 2023). A prime example is viral exit, where in HIV infection, the retroviral Gag protein must assemble at the plasma membrane to bud out and form new infectious virions. We have studied several key steps in the life-cycle of the HIV virus, including how co-factors control the Gag assembly within cells (Qian, 2023), and how timing of remodeling of the Gag lattice in budded virions is tuned for maturation (Guo, 2023). We are integrating our membrane models with our self-assembly software to model the coupled dynamics of self-assembly and budding. NSF CAREER.
Theory and modeling of non-equilibrium self-assembly
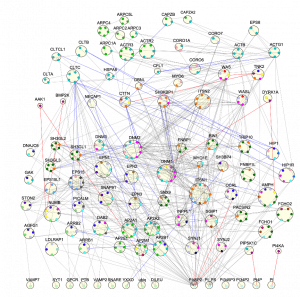
We study the mechanisms and dynamics of multi-protein self-assembly and self-organization in the cell through the development of new theoretical and computational tools. Computer simulation provides a powerful complement to experiment because we can track the spatial and temporal behavior of individual proteins at nm and sub-μs resolution, respectively, and produce a model of the collective protein dynamics. There are a number of challenges in trying to model these processes with both the efficiency to reach long (min) time-scales and the resolution to capture the structural features of a growing protein complex. We developed novel single-particle reaction-diffusion (RD) algorithms that combine both efficiency and accuracy in solving the governing equations of motion in 3D, in 2D, and transitioning from 3D to 2D (Yogurtcu et al, 2015) (Fu, 2019). These algorithms have been combined into user-friendly software (Varga, 2020) that has been applied to diverse biological systems like viral formation, clathrin-mediated endocytosis, and silk-fiber formation, with direct comparison to experiment. These simulations of complex systems also stimulate our development of simpler models amenable to analytical theory. We combined theory and kinetic models of 3-7 subunit complexes to quantify how control of assembly kinetics via optimal selection of rate constants can ensure robust and efficient assembly for multi-subunit complexes that would otherwise suffer from kinetic trapping (Jhaveri, 2024). NIH MIRA.
Dimensional reduction in biology
Proteins frequently localize to lower-dimensional environments in the cell to perform their target function, whether the effectively 2D surface of the membrane, or the 1D road of DNA. This concept of dimensional reduction was established in the 1960s as an effective means for a protein to accelerate its target search (e.g. membrane receptor), by searching in a reduced space that concentrates reactants. We have developed model systems and theory to further quantify how self-assembly, not just target searches, can dramatically benefit from dimensional reduction. By deriving an effective equilibrium constant for protein partners that can also reversibly localize to membranes, we have shown that for physiologically relevant regimes of protein concentrations, binding affinities, cell V/A ratios, and lipid populations, dimensional reduction drives dramatic increases in dimer formation, thereby regulating the initiation of robust assembly (Yogurtcu 2018). Modulating the ‘stickiness’ of the membrane via lipid populations (e.g., from enzymes) will then tune when and where assembly occurs (Varga, 2020). Somewhat surprisingly, we showed the kinetics of dimer formation can also increase by orders-of-magnitude due to 2D localization, even though diffusion on the 2D surface is ~100-fold slower than in solution (Mishra 2021). These same ideas apply to self-assembly along DNA. NIH MIRA, NIH U01.
Optimization of model parameters with super-resolution imaging data
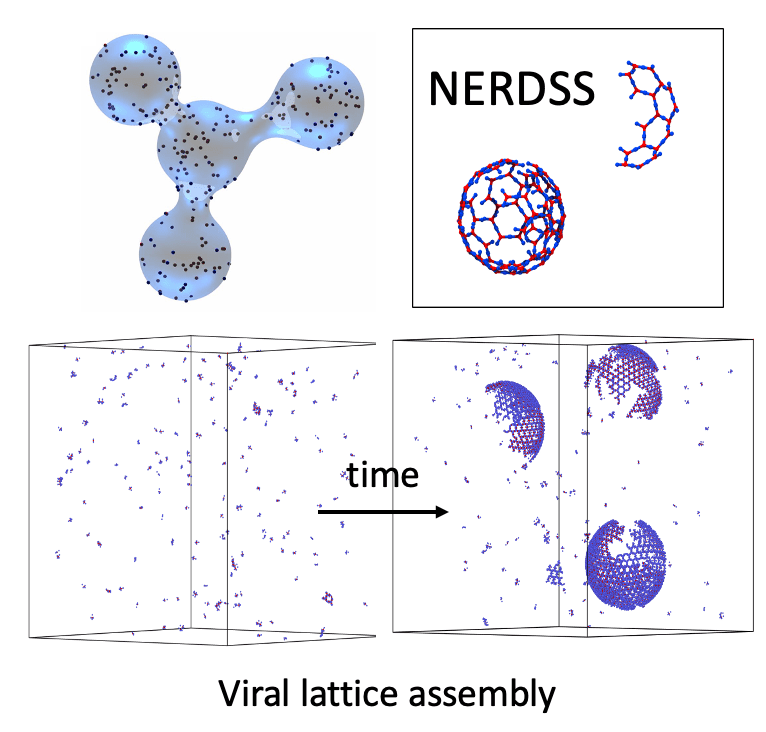
A challenge with any cell-scale biological model is defining the requisite reaction network and corresponding rate constants that can quantitatively reproduce in vivo dynamics (Johnson, 2021). We are adopting optimization methods from the machine learning community to enable searches in high-dimensional parameter spaces, similar to what is required in training a neural network (Jhaveri, 2024). A primary goal is to improve our model description as constrained by single-particle tracking data at super-resolution, which effectively monitors the same dynamics as our models, albeit typically just one molecule type at a time. We recently developed and optimized a model of dynamin clustering and assembly against live-cell sptPALM data, predicting how specific isoforms of dynamin could produce distinct densities of dynamin clusters in response to Calcium stimulation (Jiang, 2024). We are working with our colleagues at Johns Hopkins to apply our methods to quantify experimental data on self-assembly in diverse systems, including silk-fiber assembly (Xie, 2023). NIH MIRA, NIH U01.